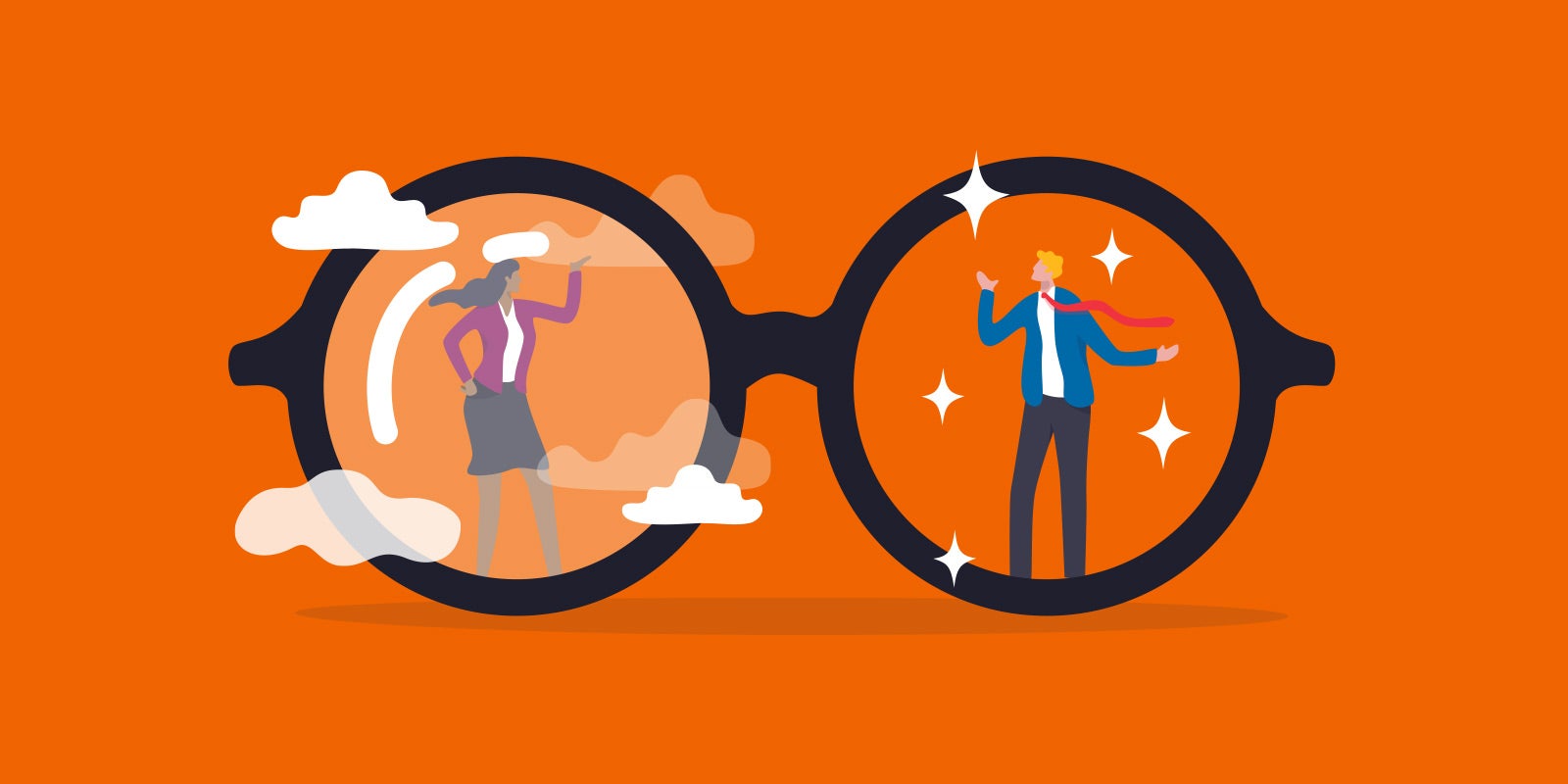
A peek into bias
Over the last year I’ve been learning different AI/ML modeling techniques and processes. My current project was a image recognition problem using Convolution Neural Networks predicting Pneumonia or Normal diagnosis in X-ray. As the main data scientist processing the data, building the model and verifying it I saw the implicit biasses and limitations of my knowledge, skills and resources creep in. The project is for educational purposes so it won’t be deployed to support my local hospital. 😅
Stewart Baker recently published an article in the Law Fare Blog entitled ” The Flawed Claims about bias in Facial Recognition“. Baker is a current lawyer in Washington D.C and former general counsel of the National Security Agency. It is safe to say, he has experience with government safety and security practices.
Baker has two main claims that stuck out to me:
- Technology is morally agnostic and it’s discrepancies shouldn’t become a moral issue. Judging technology through a moral filter, will stifle the innovation and improvement.
- Specifically with facial recognition, after understanding the real issues, there are simple technical improvements that can help bring major improvement to them .
Where the bias lies…
Moralistic Technology
I generally agree with Baker on the claim, technology itself is not always a moral issue. There are probably times when it can be, but I am not going to use this post to meditate on those times. Even though I believe there is an absolute truth on some issues, I know various people have different perspective and gradients of “morality”. We won’t dig into that either.
My main thought is, even though technology shouldn’t be judged through a moral lens, as a society we must be willing to acknowledge the current and potential (that we can reasonably foresee) applications that make it a moral issue.
Some of Baker’s examples centered around legal organizations and law enforcements use of facial recognition and the bias that occurs there. I think in most cases technology is a privilege and the ownous and responsibility isn’t on the object or tool itself, but on the users (individually and organizationally). This simple reasoning is used for a lot of other debates as well in America 🔫. I want expand on those thoughts just a little more… I think when the users of a tool do not steward it well, then the privilege to use it should be adjusted accordingly.
I am speaking in generals, so the application of these thoughts vary. In the case of facial recognition technology used within law enforcement, I have mixed thoughts. I know it has already been used, my hope is that we don’t use this tool to amplify bad practices (systemically and by individuals) that are already in place.
Systemic bias
Again, I agree with Baker’s claim that gathering more data for model training and understanding how to capture subjects better with camera will improve bias results in facial recognition.
In the field of AI/ML data is a key component to the field, the accuracy and performance of a model is highly dependent on the amount and quality of data in training.
I am glad someone is aware of the facts that historically capturing people of color was not done well. There was very little thought in understanding of how settings must be adjusted to account for the different melanin and skin tones.
I believe his solutions were correct, but limited. Facial recognition technology is not done in an isolated bubble. It is developed and deployed within a systemic context, that has bias and impacts it.
The facial recognition model that is trained and refined on data is one system (or subsystem) among the greater developed system and the application (context of deployment) of the facial recognition is it’s own system.
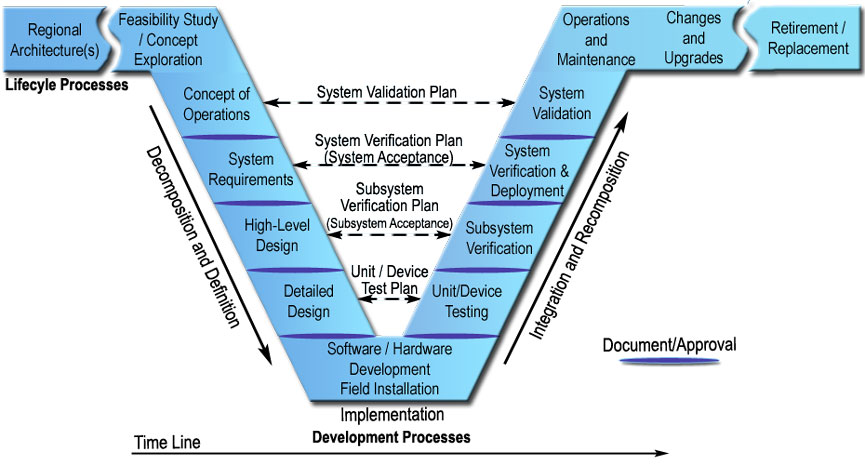
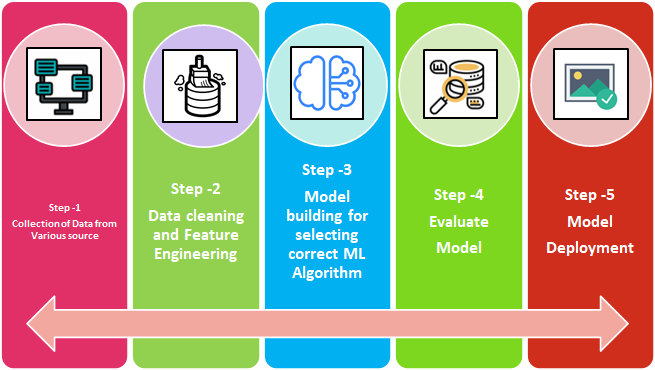
Part of a developing system is the executed by people in some major or minor role. Systems engineering is an art and a science. People bring their experience, knowledge, nuances as well as biasses into the creation of the systems.
Along with training and building a model, data collection, cleaning and feature engineering are a major part. People (along with other tools) are deciding how to manipulate the data and hyperparameters for model optimization.
In terms of people, Cade Metz‘s ,2021 article Who Is Making Sure the A.I. Machines Aren’t Racist? states “The people creating the technology are a big part of the system. If many are actively excluded from its creation, this technology will benefit a few while harming a great many.”
In the context of a facial recognition system, having different qualified ethnic, racial and genders apart of the development team is impactful. These biasses trickle into the final product. This isn’t simply because their differences are just “good” to haves. Denzel Washington said it well in an interview about his movie Fences . “Its not color, it’s culture.” Diversity in this context isn’t for a quota but will give insight into areas, that everyone just doesn’t experience.
Last, but not least deploying a facial recognition system is greatly influenced by environmental and organizational system it is applied in. The legal and law enforcement system have systemic cracks that need refactoring and healing. Adding a innovative technology could help in some areas and in some other areas further spread the cracks. Just like the previous systems I mentioned, the deployment system is full of people (with various biasses) interacting with a technology.
The closure
Edward Deming said it well,
“Every system is perfectly designed to get the results it gets.”
The facial recognition model is a system among other systems. These systems all involve people with biases. Technology biasses are apparent ; other important system influences such as people, environment and infrastructures are as well. Simple technical solutions will not solve complex deep socio-technical problems.
Legal and law enforcement agencies have a hefty task of caring and protecting people’s lives. Our society shouldn’t be looking to minimize the importance of bias but really understand where it stems from and act with the interest of people’s best interest not just technology.